Speaker Details
Training Massive-Scale AI Foundational Models on Frontier
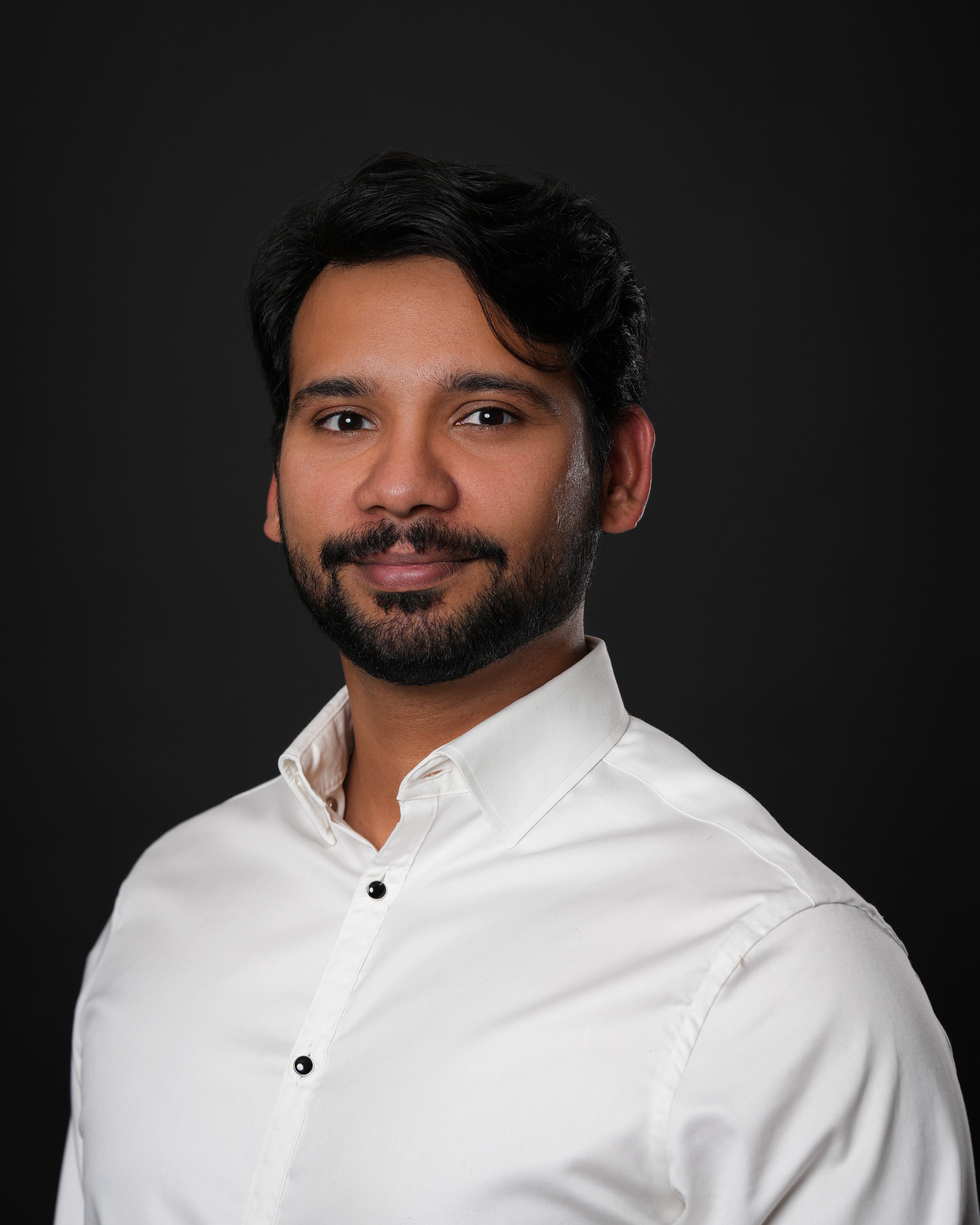
Sumanth Gudaparthi
Bio
Sumanth Gudaparthi is a researcher and a Member of the Technical Staff at AMD Research. His work explores potential acceleration opportunities in AMD roadmap devices for both large language model (LLM) training and inference, as well as applications in sparse linear algebra. He received his Ph.D. in Computer Science from the University of Utah in 2022, and his Master's degree in Very Large-Scale Integrated Circuits (VLSI) from IIIT-Hyderabad, India, in 2015. His research has been published in several prestigious venues, including MICRO, HPCA, ASPLOS, S&P, and TNANO. He has also served as a program committee member for numerous notable conferences such as ISCA, HPCA, ASPLOS, and IPDPS. Before pursuing his Ph.D., he worked as a design engineer at AMD, where he focused on client SoC system security verification and debugging.
Abstract
AI foundational models are poised to accelerate scientific discoveries and revolutionize the way we conduct scientific research. The fast-changing AI landscape with ever larger model sizes and model complexities is making insatiable demands for supercomputer-class systems for training and deployment of these AI foundational models. In this talk, we will discuss our experiences of working with a multidisciplinary team of researchers, engineers, and scientists from AMD and ORNL to train some of the world's largest AI foundational models with millions to billions of parameters on Frontier, the world's current fastest supercomputer. Specifically, we will discuss how we trained: (1) Large language models (LLMs) for scientific research, (2) Graph neural network (GNN) foundational models for material discovery, and (3) advanced vision-transformer-based climate AI models for Earth system predictability. Our talk will include topics ranging from efficacy of AI foundational models, scale-up challenges and solutions, model and data partitioning strategies, performance monitoring tools at scale, comparison with state-of-the-art models, and future research directions.